6 steps to facilitate data-driven decisions and unleash innovation

Going by your gut feeling or using data to make decisions are two very different ways of doing things, despite the fact that they might both lead you to the same result. Data-driven and goal-driven are opposite sides of the same coin and they tend to work best when they complement each other. Before delving into the distinction between the two, let’s begin by understanding a fundamental data transformation process.
The DIKW Model
Statistical models, machine learning, artificial intelligence, collective human intelligence... What these have in common is a very general underlying data transformation process, called “The DIKW Hierarchy”:
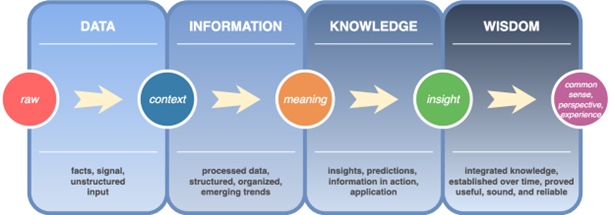
As the diagram shows, DIKW stands for data, information, knowledge and wisdom - it all begins with data. Data collection, storage, retrieval, manipulation, visualization, and other types of processing are the first steps to preparing raw input. This is usually done via sensors, detectors, and sometimes even human senses. Once collected, the next step is analysis which means looking for patterns. It requires following a strict sequence of steps, otherwise, you risk drawing incorrect conclusions which could result in wasting time and resources on wishful thinking. Extracting patterns from the data implies organizing and transforming data into information - now we have something to work with. Information itself can be considered structured, stationary context into data.
This very text that you are reading contains information. Do you find it useful? Are you considering applying some of that information to your own use case? If the answer is yes, then this is one very simplistic example of using information and turning it into knowledge. To put it plainly, knowledge is information that serves a purpose or is practically applied. Last but not least, wisdom is the last stage of this process and represents accumulated and integrated knowledge that has proved correct. It usually manifests over long periods of time and is associated with experience. Wisdom is what could eventually shape society and culture. Whilst immensely important, there is no clear shortcut to wisdom, which however does not stop us from reaping the rewards of the first three stages.
Goal-directed nature
You may argue that the aforementioned process does not entirely align with how we humans process data and you will be partially correct - we can be much more chaotic and impulsive when it comes to decision-making. Humans are mainly driven by very specific goals, which is closely linked to our biological and somewhat irrational nature. This results in skipping some of the steps above, reordering the model, introducing bias or just making reckless choices. The same goes for other animals and living systems - practically any agent that pursues a direct objective, relying on immediate environmental cues and intuition. This is nature’s way. Natural selection has been set in motion on planet Earth almost 3.7 billion years ago. Its primary goal is successful reproduction and its main tool - error. While nature’s brute-force way of finding optimal solutions took millions of years, we humans don’t have this luxury of time.
Moreover, in an operational innovation business, there is a very limited margin for error. Of course, making such is inevitable (and sometimes necessary), however, you can have goal-oriented targets met with the help of informed, data-driven decisions. What this means is having the right tools to purposely steer clear of predictable mistakes and avoid sub-optimal actions or conclusions.
Data as a catalyst
How is success measured? What is a quintessential component leading to bigger impact? Innovation. This is where data comes into play. You have probably heard the expression “data is the new oil”. We can modify this slightly by saying that data is the new fuel and innovation thrives when fueled by data. All this may seem straightforward, but how to use that data to your advantage is what’s not immediately obvious. The reason is that this process involves a very complex statistical and mathematical approach, as well as robust data infrastructure, skilled analysts, and a data-driven company culture. Furthermore, innovation is not a one-time event but a continuous process of improvement and adaptation. Data-driven decision-making provides the feedback loop necessary for iterative innovation by reducing uncertainty and mitigating risks. Instead of relying solely on intuition or guesswork, organizations can utilize data to validate assumptions, test hypotheses, and make evidence-based decisions. This leads to more efficient allocation of resources and increased innovation ROI.
6-step guideline for facilitating data-driven innovation
Step 1: Data Collection and Exploration
The first step in the data-driven decision-making process is to gather and explore relevant data. This data can come from various sources such as customer feedback, market research, industry reports, and internal performance metrics.
- Identify the Right Data Sets: Understand what data is relevant to your business objectives and where to find it.
- Ensure Data Quality: Cleanse and preprocess your data to ensure it's accurate, complete, and reliable.
- Organize the Data: Structure your data in a way that it can be easily analyzed. This might involve creating databases or using data management tools.
Step 2: Analysis and Insight Generation
Once you've collected and organized your data, the next step is to analyze it and generate insights.
- Apply Analytical Techniques: Use statistical analysis, data mining, and machine learning algorithms to extract meaningful insights from your data.
- Explore Patterns and Trends: Look for correlations, trends, and patterns that can inform your decision-making process.
- Visualize Your Data: Use data visualizations and dashboards to communicate insights effectively. This can help stakeholders understand the data and its implications.
Step 3: Ideation and Concept Development
With the insights generated from your data, you can now start the ideation and concept development process.
- Engage Cross-Functional Teams: Involve designers, engineers, marketers, and business strategists in brainstorming sessions to generate innovative ideas.
- Align Ideas with Opportunities: Ensure that the ideas generated align with the identified opportunities and market demands.
Step 4: Prototyping and Testing
After developing your concepts, it's time to translate them into tangible prototypes or minimum viable products (MVPs).
- Create Prototypes or MVPs: Develop tangible representations of your ideas for testing and validation.
- Conduct Rapid Testing: Gather feedback from users, customers, or relevant stakeholders through testing and experimentation.
- Refine Concepts: Use the feedback to uncover potential issues and refine your concepts.
Step 5: Iterative Refinement and Optimization
The next step is to refine and optimize your prototypes or MVPs based on the feedback received.
- Incorporate Feedback: Make necessary adjustments to the design, features, user experience, and performance based on user preferences and identified areas of improvement.
- Continuously Test and Refine: Keep testing and refining your product to ensure it aligns with customer needs and expectations.
Step 6: Implementation and Scaling
Once your innovation has been thoroughly tested, validated, and optimized, it's time to scale up.
- Develop a Comprehensive Plan: Create a detailed plan for production, marketing, or implementation. This should consider factors like resource allocation, supply chain management, marketing campaigns, and stakeholder engagement.
- Scale Up: Implement your innovation on a larger scale, whether that means bringing a product to market or integrating a new process within your organization.
Implementing data-driven decision making – A practical example
Let’s continue with an example of an innovative product, which would have been impossible to develop if not driven by data. The COVID-19 pandemic proved a formidable challenge to everyone. Among everything else, you might recall that one of the biggest concerns for every country was the test on its local health system and the hospitals. Thankfully, we had the means to seek and gather as much data as possible on the problem. With the use of such data, we were able to develop a solution for a customer which is focused on the prediction of Coronavirus hospitalizations 14 days in advance.
The problem is called Time Series Forecasting and requires large amounts of input. Open-source datasets from the Robert Koch Institute were utilized, which include numerous data points such as infection rate, age, vaccinations, lockdown periods, death rate, etc... After the raw data has been processed and meticulously analyzed, certain patterns were identified and very concrete features were selected for prediction. With the already processed data at hand, a Linear Tree Regression model was applied for prediction, which is a type of Machine Learning algorithm. The point here is not solely to predict hospitalizations per se but to help hospitals prepare in advance by stocking up on supplies for future COVID infection surges.
This means:
- optimizing costs for both materials and staff
- avoiding unnecessary stress due to limited time and capacity
- last but not least - help save human lives

Data is your superpower
There may not have been a better time to digitize your business and make use of data to drive your goals and innovation forward. How? We can help you with that. Why? Three reasons:
- Most companies that don’t use data to their advantage will become companies of the past
- Now more than ever we understand how to handle data of any size
- Artificial Intelligence is here to stay - becoming data-centric is the first step towards incorporating it into your business
Being data-driven means taking higher-resolution snapshots of current reality, which when combined, could paint a picture of what lies ahead. From doing predictive maintenance to saving human lives, using data to your advantage is no easy task. Nevertheless, it could provide more knowledge, which means more control and effective decisions on the path to achieving your business goals. In an era where everybody talks about AI, we need to get back to data first.
References: Ackoff, R. L. (1989). From Data to Wisdom Journal of Applies Systems Analysis 16, 3-9. Ackoff RL.
Let's connect!

Subscribe to receive the latest insights directly to your inbox.